- Tags:: #📚Books, [[Data methodology]], [[Data Science]], [[My engineering management principles, values, and practices]]
- Author:: [[Jike Chong]] and [[Yue Cathy Chang]]
- Liked:: #3/5
- Link:: [How to Lead in Data Science (manning.com)](https://www.manning.com/books/how-to-lead-in-data-science)
- Source date:: [[2021-12-19]]
- Read date:: [[2021-11-01]]
- Cover::
![[cover_how_to_lead_in_ds.png|100]]
Los autores no los conocía antes, pero tienen parece que tienen background bastante largo y bueno en DS a nivel ejecutivo (e.g., Jike Chong ha sido Data Science Director en LinkedIn). El libro sería un [[📖 The Manager's Path]] del Data Science pero más tocho.
Es práctico hasta la nausea. Prácticamente todos los capítulos tienen tablas muy extensas de auto-evaluación con las habilidades que has de tener y cómo desarrollarlas.
Muchos de estos libros son un extenso compendio del sentido común y corren el riesgo de ser poca mantequilla en una rebanada de pan muy grande. Pero, ahí está el quid de la cuestión, la diferencia entre conocimiento y sabiduría: hay que pegarse **ese trabajo sistemático de trabajarse cada punto, de cristalizarlo.**
**¿Hay sorpresas en el libro? Yo diría que no a grandes rasgos. Las cagadas que se cometen en Data Science son clichés.** La vida es una sucesión de lugares comunes. Un rollazo muchas de las veces, vaya. Pero las seguimos cometiendo una y otra vez. Esto es claramente [[✍️ Refusing to stand on the shoulders of giants]]. Es decir: Data Science es un campo con un porcentaje de fracaso alto, con mucha incertidumbre, hay que ir a simplificar, tener pies de plomo con las conclusiones que uno saca, tener quick wins antes de ir a por proyectos más ambiciosos, comunicando mucho a los stakeholders, tiene que haber entendimiento, colaboración y apoyo por parte de los mismos...
Ahora, sí que me hace gracia que hay un foco muy fuerte en el libro, en distintos lugares, hacia casi la autoayuda para que mantengas una actitud positiva. Entre la dificultad intrínseca del campo, y la complicación a la hora de relacionarte con el resto de la organización, es difícil no frustrarse.
## 1. What's in a successful data scientist?
### Esto es muy nuevo todavía:
>95% of the companies with data science teams have teams of fewer than ten team members. (p. 1)
>The field is still nascent that leadership talents who can lead projects, nurture teams, govern functions, and inspire industries are scarce and in high demand (p. 1)
De 2010... La capacidad de usar paquetes de Data Science sin tener claro lo que pasa por debajo.
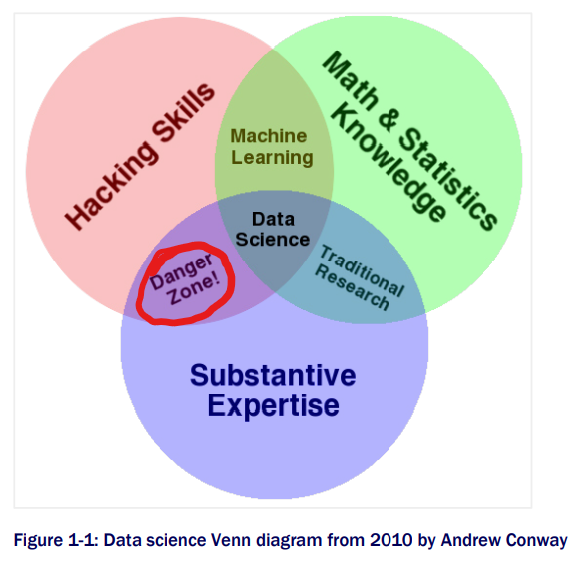
### Qué deberíamos tener
Ese diagrama consideran que ha evolucionado a dominio tecnológico, capacidades de ejecución, y conocimiento de experto (que luego aclaran que sobre todo es que te muevas como una culebrilla por la organización). Le añaden 3 virtudes y con eso construyen el abanico TEE-ERA. Disco, Ibiza, Locomía:
- 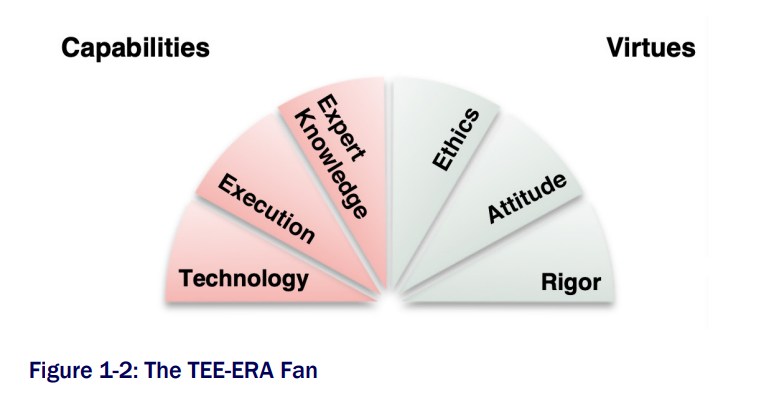
Define las 3 virtudes del data scientist como: ética, rigor y actitud (positiva), pero lo que más me gusta es que:
>Virtues are meant to be practiced in moderation. Doing too much is just as bad as not doing enough. For example, too much rigor can cause analysis-paralysis ([[paralysis by analysis]]) and indecision. (p. 3)
El libro es casi como de **autoayuda**. No deja de hacer mucho énfasis en que mantengas una actitud positiva y no te frustres porque data es difícil, y que te tomes desayunos con tus compañeros para que tu equipo no acabe alienadísimo. ^95c239
>Technically strong, Paul also invests significant effort in building relationships with the data science team. In addition to project-related meetings, Paul sets up weekly “walks” with each of the seven team members, holds weekly office hours to make sure he is available for the team, especially when challenges arise, and hosts breakfast-with-data-science in an effort to communicate frequently with project stakeholders and business partners to listen to their needs and keep them informed. (p. 8)
En el debate [[Generalist vs specialist]], la obviedad: que al inicio de un equipo de Data es más fácil aportar valor siendo generalista, pero que con el tiempo necesitarás t-shaped con distintas expertises (p. 5).
## 2. Capabilities for leading projects
### Framing the problem (p. 19)
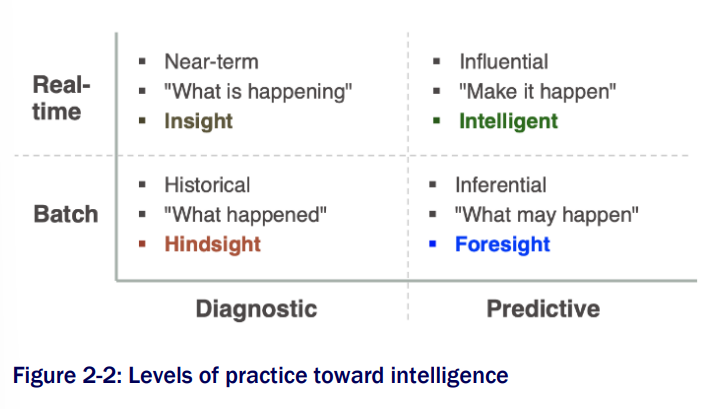
Que hay que tener **mucho cuidado** con las conclusiones que se sacan de un análisis: problemas de sample size, data sparsity, outliers, sample imbalance... Todo este **potajeo**.
El típico consejo de que vayas a lo **simple**:
>In many use cases, however, because of the small sample sizes, labeling difficulties, and interpretability and operability considerations, you may opt to use simpler models and engineered features to discover patterns in the scenarios (p. 22)
Elegir qué modelo usar es táctica, lo interesante es "cómo nos ponemos a construir modelos" que es estrategia (p. 25). En orden de cómo irías aplicando distintos tipos de algoritmo:
- Reglas básicas (nada de ML).
- Reflexive: cuando es complicado definir una frontera entre casos positivos y negativos y tenemos que explorarla. E.g., en antifraude, marcar casos sospechosos para revisión manual.
- Momentum-based (inertia-based): capturar tendencias, señales que puedes cuantificar y en un momento dado, actuar.
- Foundational: cuando ya puedes modelar correlación o causalidad para predecir el outcome.
Búscate proyectos en la organización que sean, importantes, útiles, y merezcan la pena (ROI) (p. 47).
Project management: Scrum en Data Science? It depends!
>Smaller data science projects with clear definitions can be well-suited for the scrum process with minimal planning overhead. More extensive data science projects with many failure modes often require a light-weight planning and alignment process. Project phases are defined to take advantage of scrum processes that can adjust to the specific learning from phase to phase (p. 41)
### Las capabilities de Data Science, quiénlasconoce
Si según Gartner fracasan el 8% de los proyectos de Data Science, no es por lo que crees:
>Many of the failures are caused by not the lack of technological prowess, but the quality of the execution. There are three major stumbling blocks in data science project execution:
• Specifying projects from vague requirements and prioritize.
• Planning and managing a data science project for success.
• Striking a balance among hard trade-offs.
- La madre del cordero (p. 31):
>**Interpreting business needs can be challenging for new data science tech leads. Many of your partners are not yet familiar with all the capabilities data science can bring and provide requests that at best may be a sub-optimal framing of the problem**.
>Compared to other technical or engineering projects, data science projects often involve more partners, face greater uncertainties, and are **harder to manage for success.**
## 3. Virtues for leading projects
### Rigor
Otro cliché:
>For many data scientists, exposing simplifying assumptions in an initial version of a project may feel embarrassing and not rigorous. As tech leads, we should all have the humility and understanding that assumptions are necessary and essential to prioritize our time on our analysis’s most important and sensitive factors. (p. 69)
Me hace gracia leer esto, porque es verdad, pero hay un colorario: también vas a recibir presiones para NO ser riguroso:
>Partners expect our work to be scientifically rigorous, and the responsibility of holding up that bar of rigor is in the hands of the data science tech leads: in your hands. (p. 70)
The foundation of scientific rigor:
>ensure resources are dedicated to the most promising endeavors in the most efficient manner.
>Executing experiments: This is the core of the scientific method of crafting the hypothesis, testing it, and iterating until impactful learning can be validated. (p. 70)
>The rigor of the scientific methods is taugh less frequently, and in some aspects, need to be practiced rather than taught. As a tech lead, you should be sensitive to these background differences and gently guide data scientist (nota mía: y a la organización entera!)
Claves del rigor:
>1. Redundancy in Experimental Design
>2. Sound statistical analysis
>3. Recognition of error
>4. Avoidance of logical traps
>5. Intellectual honesty (p. 71)
Aquí el peligro es la increíble accesibilidad de métodos estadísticos, ML, etc. pero al mismo tiempo la inaccesibilidad de correctas interpetaciones, cuando tenemos más de 175 sesgos cognitivos posibles, y cosas tan divertidas como la [[Simpsons paradox]].
El quinto punto es brutal:
> Intellectual honesty is a mindset in scientific rigor. It is the acknowledgment of nagging details that do not fit with one’s hypothesis (p. 74)
Es muy fácil conectar esto con lo que se dice en [[📖 Noise. A Flaw in Human Judgement]]:
>The only measure if cognitive style or personality that they found to predict forecasting performance was (...) developer by psychology professor Jonathan Baron to measure "[[actively open-minded thinking]]." To be actively open-minded is to actively search for information that contradicts your preexisting hypotheses (...). They disagree with the proposition that (...) "intuition is the best guide in making decisions." (p. 234)
### Actitud
Take responsbility for entreprise value
>1. Clarity on Goals: Move business metrics with projects using the simplest method.
>2. Focus on Velocity: Succeed quickly by failing fast.
>3. Communicate the impacts: Listen, engage, and lead.
La clave del velocity es: speed to initial solution and low friction to incremental improvements. PEEEERO:
>Low friction to incremental improvements requires strategic choices and timely resolution of technical debts such that there is a firm foundational architecture for iterative innovation (p. 81)
Esto es complicado de vender: que para ir deprisa... tienes que hacer una inversión upfront que no está nada mal. Como luego dicen:
>Most data science projects are building tracking and data foundations, servicing technical debts and/or supporting daily business operations.
>1. Tracking Specification Definition
>2. Monitoring and Roll-out
>3. Metrics Definition and Dashboarding
>4. Data Insights and Deep Dives => direct data-science-driven business impact
>5. Modeling and API Development => direct data-science-driven business impact
>6. Data Enrichment
>7. Data Consistency
>8. Infrastructure Improvements
>9. Regulatory Items
Of the two projects types with direct data-science-driven business impact, Data Insights and Deep Dives are recommending new features or process recommendations.
Más cosas que son complicadas de vender en una organización principalmente de software, directos de [[🗞 Hidden Technical Debt in Machine Learning Systems]]:
- Que los modelos son máquinas de acoplar
- El CARE principle: "Changing Anything Retrain Everything".
La parte de autoayuda:
>Winston Churchill once said: “Success consists of going from failure to failure without loss of enthusiasm.”
>
>**Data science is a field with high failure rates. It is common to expect 70% of the experiments not to show positive results.** In well-optimized domains like Bing, Google, and Netflix, success measures are about 10-20%. It takes an immense amount of curiosity and tenacity to stay upbeat and focused on staying the course to deliver project wins.
Pero aquí está la importancia de comunicar:
>Being a tech lead who manages data science projects can be challenging. When the team is performing well and enjoying their work, it can feel incredibly rewarding for you. When deadlines are looming, or when technical debts become overwhelming, the situation can feel quite stressful. Leading is not just in the technical area, but also in the virtues and attitude to facilitate a productive work environment. One technique for motivating the team and building trust with partners as the tech lead is to communicate any institutional learning from succeeded and failed projects promptly and regularly. These crystallized learning can help individual team members stay motivated in their projects by recognizing their impact on the business, and help your business partners and executives see the progress toward bigger wins down the road. (p. 94)
### Respect Diverse Perspectives in Lateral Collaborations.
Esta me pilla en un punto justo interesante. Te piden un algoritmo de predicción de venta PERO, va a haber actuación constante sobre la esa venta de distintas maneras.
>Data science practitioners may gasp in this case, as sales strategy and compensation adjustments are nightmare situations for the predictability of a revenue model (...) when the sales strategy and compensation plan change, the predictions may no longer be relevant. (p. 97)
> The global alignment may look more troublesome and less appealing from the perspective of efficiently completing a project. However, from an enterprise perspective, sales strategies and sales compensation will be adjusted from time to time. It is better to be part of that process than build a model based on historical data and be out-of-date in a quarter or two.
## 4. Capabilities for leading people
Es curioso que te recomiende que como manager, tu te quedes con un big bet y todo el junk y deleges lo demás: serás el que está en mejor posición para redefinir un proyecto o cancelarlo.
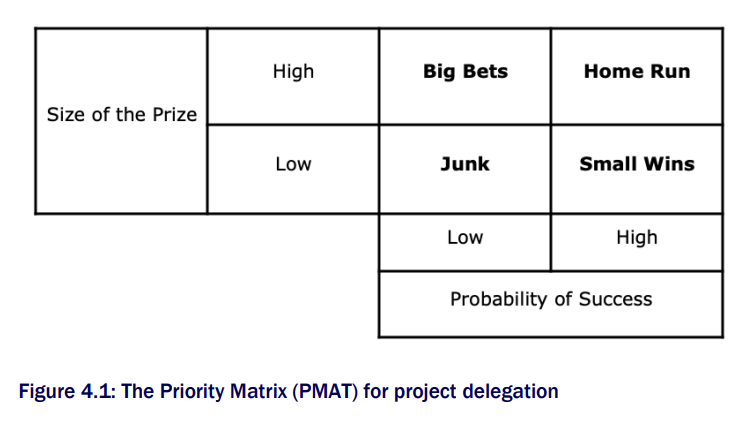
Para [[SWE hiring best practices]]:
>For roles that will frequently be interacting with business partners, it is critical to set up an interview panel that includes those partners in products (p. 115).
Que tienes que controlar de negocio, claro...
>You can check out the blog [16 Startup Metrics | Andreessen Horowitz (a16z.com)](https://a16z.com/2015/08/21/16-metrics/) from the Venture Capital firm Andreessen Horowitz for more business metrics discussions (p. 133)
porque si no, no identificas oportunidades
>As the manager of a data science team, your team looks up to you to connect data science capabilities to company priorities. Your responsibilities require you to think beyond the business partners’ requests to discover fundamental data opportunities that only someone with deep data science expertise can recognize.
Assess ROI:
>This is the key difference between intelligence and wisdom. Intelligence is the ability to make good decisions with complete information. Wisdom is the ability to make good decisions with incomplete information. (p. 136)
En cuanto a impacto para evaluar en un RICE, dado que es difícil evaluar en un proyecto de data:
>In the absence of experience from a similar project, you can reverse the question to what magnitude of improvement is required to make a project worthwhile? (p. 137)
## 5. Virtues for leading people
### Represent Team Confidently in Cross-functional Discussions
Buenísima esta, es cierto. También te digo que la gente en general subestima la capacidad de comprensión de otra gente.
> You may have seen some drafts of junior data scientists’ presentations. In an attempt to be rigorous, it starts with 10min of experiment setup and caveats, followed by an initial result, and ends with many technical uncertainties in the analysis. Such a presentation opens up more questions than answers, leaves the audience feeling more confused than before, and does not generate much trust with the cross-functional business partners. Data science results are hard to present as there are many uncertainties and caveats in any one analysis. However, **the responsibility of data scientists is to answer questions and not to cause confusion** (p. 149)
¿Cómo arreglar esto? Nos propone dos vías simultáneas:
a) Strong opinions, weakly held: partir de una hipótesis fuerte al principio e ir revisando.
b) Storytelling:
- Habla de lo que le preocupa a tu audiencia.
- Da recomendaciones accionables.
- Buena estructura: menos es más.
Pero claro, cuando no está tan claro lo que recomendar...
>If there is contradictory evidence, we should stop crafting the presentation and go back to forming a different hypothesis that is consistent with the available evidence (p. 151)
O bien:
>You can also present multiple options you may have considered in the “strong opinions, weakly held” exploration process and highlight the trade-offs between the different options.
### Drive Clarity in Distilling Complex Issues into Concise Narratives
También buenísima esta.
>In your work, you may face many complexities in technical and business situations. **You are given more responsibilities to manage a team because of your ability to comprehend significant complexity. But others may not be as skilled in handling complexity.** Now is the time to hone your craft at simplifying the complexities you encounter in your work (p. 164)
>You also have the responsibility to produce a culture of learning institutionalization (p. 175)
Ojo porque los post-mortems, no basta con hacerlos... hay que recordarlos.
>In a fast-growing organization, it is not enough to document post-mortems for incidents and archive them. They need to be reviewed regularly. Well-written post-mortems are like business cases (p. 176)
## 6. Capabilities for leading a function
Es bastante fuerte notar el hincapié en que tienes que ser optimista porque está difícil:
>You may run into negative people with negative comments. Focus on the positive partnerships and be patient in bringing people in the negative partnerships around over time. Be optimistic, hopeful in working through organizational or technical roadblocks. This is key to maintaining the energy you need in the project to see it through (p. 196)
Otro clásico. Pero hay gente que esto lo lleva demasiado lejos...
>Great leaders are not perfect data scientists. They leverage their team to compensate for their personal weaker area. In the end, it is the output of the teams that speak for the effectiveness of the leader. (p. 206)
Ojito al [[SWE hiring best practices]]. Por un lado dice:
>When your talent brand is not as strong, requesting a take-home case study at the beginning may dissuade potentially strong candidates (p. 211)
Pero luego, cágate:
>Technical interviews are usually organized as a sequence of three to four interviews of 45-60 minutes in length. Each interview should cover one or two essential areas the team is hiring for.
Yo aquí la verdad que mi opinión la tengo clara [[✍️ GPT-3 me va a quitar el trabajo, pero yo tengo que estar entrenando algoritmia de bajo nivel]].
**Tu primer equipo es... los otros leaders!**
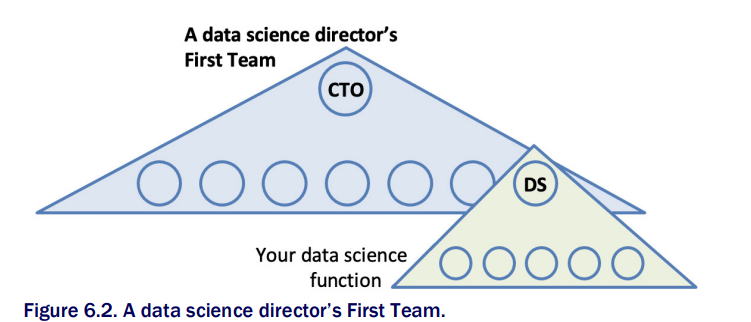
> Data science is a highly collaborative field. When you as the function leader do not coordinate well with your first team and align company or organizational priorities with partner functions, your team members suffer the most as they attempt to do their job without the support of partner team leaders (p. 215)
Me sorprende un poco porque mi sensación aquí es que mi primer equipo debería ser los leaders de fuera de ingeniería (producto, Negocio, diseño...)
## 7. Virtues for leading a function
### Ser el Relaciones Públicas de Data Science
Como cuando buscas en Tinder:
>As a leader of the data science function, identifying projects with more stable sakeholders can reduce stakeholder risks. Your responsibilities include nurturing stakeholder relationships, monitoring the project's priority, and following its impact on the stakeholder (p. 236)
### Coach as a Social Leader with Interpretations, Narratives, and Requests.
>Your leadership practices also need to transition from one of individual leadership to that of social leadership.
>Social leaders lead by offering interpretations of the situation, narratives for direction, and requests for coordinated action.
Esta "hits close":
>At the director level, you may not be aware of all the coordination details required for success. The common symptom of this failure mode is when **the team achieves the KPIs you laid out, but function fails to produce the business impacts** (p. 238).
### Rigorous planning, higher standards
Cuando te das cuenta de que todo es un cliché:
>You may have experienced or observed extremes of the planning process where it is conducted bottom-up. There is often a lack of focus where the function ends up **chasing more priorities than data scientists**. This lack of focus can result in important projects failing to get sufficient resources. At the other extreme, when a planning process is driven top/down, the resulting plan can demand unrealistic goals and alienate key players, as their perspectives and expertise are not taken into account (p. 245).
Planeando en la incertidumbre:
>A successful and rigorous planning process achieves three goals: highlight priorities, set realistic goals, and leave flexibility in execution. The best annual plan is not the most detailed. In data science, many of the issues, roadblocks, and insights are not yet known during the planning process. Anticipating them in planning means** including flexibilities in a realistic delivery schedule** such that teams and partners can align on expectations (p. 245)
¿Qué proceso de planificación sugiere esta gente? Pues..
1. Contexto. Tienes que aclarar con los executives lo siguiente, **por escrito**.
> 1. Vision and Mission: The desired future position and its approach to getting there.
> 2. Goals: Specific results over a specific time horizon
> 3. Strategies: The path to the goal within the time horizon
> 4. Strategic Pillars: Three to five top priority bets, each includes the following.
> a) Description: What is the bet?
> b) Meaning: What if we don’t achieve it? What will success look like?
> c) Key initiatives: Distinct track of work for achieving success.
2. Los equipos responden con propuestas dentro de las "bets"
3. Integración: los ejecutivos reciben las propuestas, priorizan y se integran en una estrategia coherente.
4. Buy-in: se comparte esa estrategia con todo el mundo, y se tratan aquellos flecos que hayan quedado.
## 8. Capabilities for leading a company
(__Intentionally left blank__) Me ha parecido un capítulo demasiado genérico como para que me aportara nada.
## 9. Virtues for leading a company
### Rigorous leading, higher standards.
De nuevo, self-help in Data:
>A productive and harmonious work environment can be challenging to create. (p. 327)
Entiendo perfectamente esto:
>Being able to make hard calls rigorously based on limited information with velocity is an essential skill of a data science executive.
y me recuerda a [[A method for measuring analytical work]].
Pero no estoy tan de acuerdo con esto, o al menos ese "conviction" debería ser muy "nuanced":
>you are also expected to make rigorous decisions with speed and conviction (...) being indecisive can miss opportunities and cast doubt on executives' leadership capabilitites.
Como dicen en [[📖 Noise. A Flaw in Human Judgement]], un buen lider no se parece al líder súper convencido que le gusta a la gente:
>The personality of people with excellent judgment may not fit the generally accepted stereotype of a decisive leader. People often tend to trust and like leaders who are firm and clear and who seem to know, immediately and deep in their bones, what is right. Such leaders inspire confidence. But the evidence suggests that if the goal is to reduce error, it is better for leaders (and others) to remain open to counterarguments and to know that they might be wrong. If they end up being decisive, it is at the end of a process, not at the start.
Dos causes de no alineamiento entre data y otros ejecutivos: falta de confianza y falta de entendimiento.
- **En falta de confianza:**
- Que trates las colaboraciones como **trabajo entre iguales**, o los otros equipos las entenderán como "historically mismanaged operating state saved by clever data science".
- Que los mantengas bien cerca, durante todo el proyecto (participando en todo, bien informados...)
- **Necesitas un lighting-rod:**
> you will inevitably step into some sensitive situations. To keep trust-building on track, you will need a powerful figure in the organization as a “lighting-rod” to absorb any attacks when attacks come your way. Examples of powerful figures in the organization can be your projects’ sponsors, champions, or the CEO (p. 330)
- En falta de entendimiento:
- Subestimación de las capacidades, es decir, que solo hagas dashboards.
- Sobre estimación: que la gente se crea que haces magia.
- Que roadmapes muchísimo a los ejecutivos para transmitirles lo que es realista alcanzar con lo que tengas en ese momento.
- Que con la cantidad de problemas de data quality que habrá, que priorices muy bien y no mueras por trabajo ad-hoc.
## 10. Landscape, Organization, Opportunity, and Practice
### Organization
Hay muchas maneras de organizar un equipo de data... pero si no es "equipo de Data", pues...
>When a data scientist is a member of a non-data function, they are often in supporting roles with unclear career growth paths. When companies commit to building a data function, professional growth paths for data scientists can be more clear. (p. 368)
Por otro lado, si haces un "equipo de Data", tienes, entre otros, dos riesgos importantes:
>Becoming isolated from the real business cases in partner functions (...). Mitigating techniques include identifying and producing quick wins and staying close to business needs first before committing to larger infrastructural projects (p. 369).
>Becoming an executive consulting branch for investigating ad hoc business questions: priorities are focused on the urgent, immediate concerns rather than the strategically essential bets. Mitigating techniques include identifying strategic business opportunities, crafting roadmaps, and aligning priorities with the rest of the executive team for early wins that carry the company forward on a more strategic path (p. 369).
### The Opportunity
Aquí el dominio del problema es aún más importante que en Ingeniería:
>A significant component of your leadership capability comes from the deep industry expertise you can develop on the job. (p. 375)
>When selecting an industry to pursue, it should be one that you can be passionate about. **It can take two to five years to build up expert knowledge in an industry** (p. 377)
En una empresa, el curro de Data Science va a depender de la fase en la que está. Si estás en fase de crecimiento, pues sobretodo análisis (ROIs, adopciones de features...) y optimización de adiquisición de usuarios. Si es madura...
> revenue optimization, retention, and feature adoption. One particularly impactful effort is to operate a robust A/B test infrastructure with high precision to measure incremental improvement in key metrics. In a mature business with broad reach, even marginal improvements of 0.5% in key metrics can significantly impact revenue (p. 379).
Y a incrementar la ventaja competitiva que tengas.
## 11. Leading in Data Science and a future outlook.
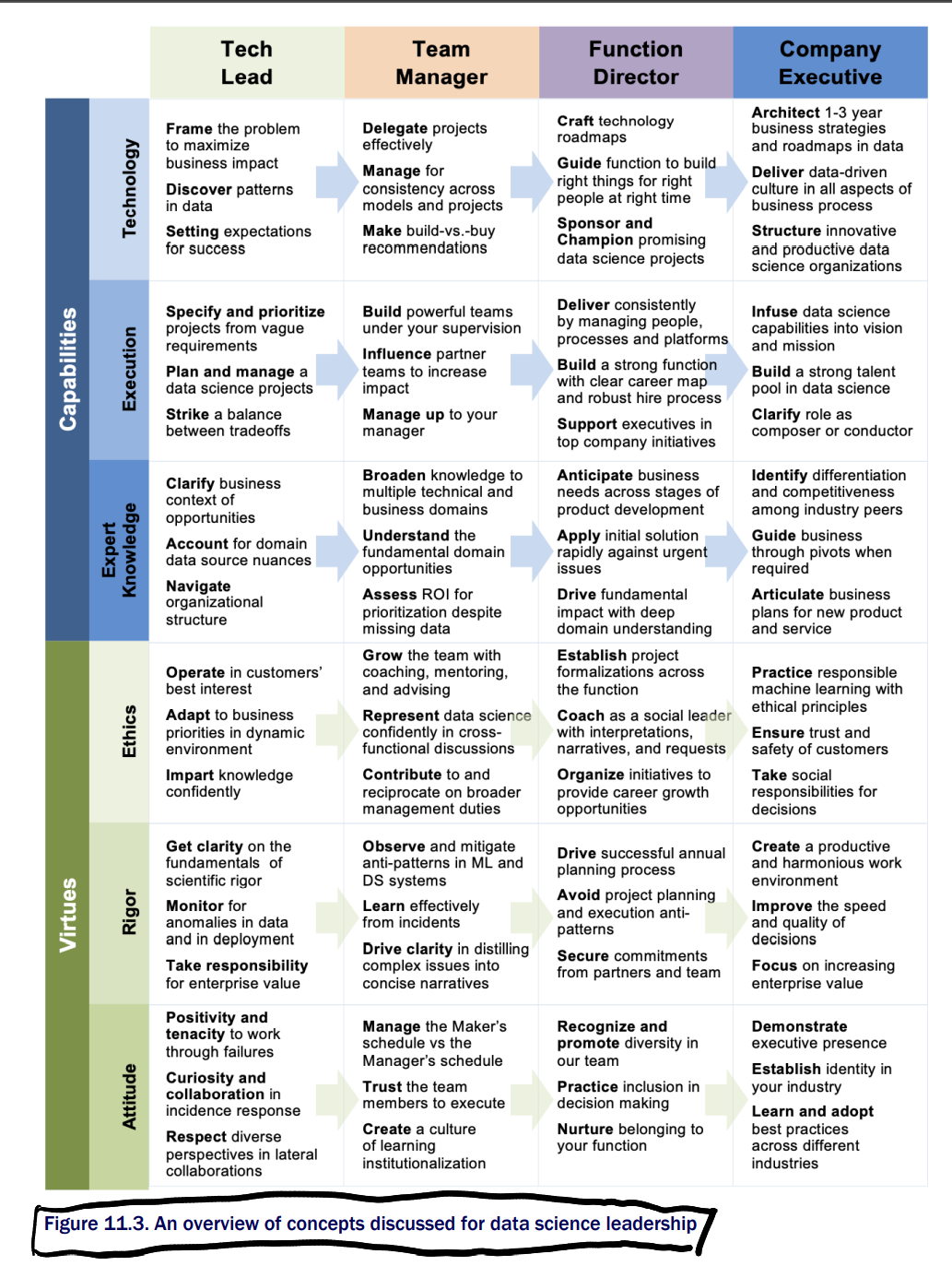
En el típico debate [[nature vs nurture]], me ha gustado mucho esto que resaltan del [[Confucianismo]]:
>Confucianism teaches that all people are capable of learning and that failure is not a result of a lack of ability but a lack of effort. (p. 415)
Y especialmente esto otro:
> One core concept from Confucianism teaching is the career moves a person can make toward a peaceful and happy world. Applied to practicing data science, it speaks to inspiring your industry with the innovation you produce. To get there, there are a total of eight steps:
1. Discover the operating principles (格物)
2. Be disciplined in getting to the heart of the truth (致知)
3. Be principled in the standard of conduct (诚意)
4. Maintain moods of positivity, modesty, and respect (正心)
5. Cultivate one’s leadership skills (修身)
6. Nurture a team (齐家)
7. Direct a function (治国)
8. Inspire an industry (平天下)(p. 416)
### The Future Outlook: nos faltan PMs de Data
Nos faltan data product managers. Que simplemente es [[data literacy]] en product managers. Mientras tanto, son los DS los que están navegando ese gap.
>The scarcity of data product manager talent is a significant bottleneck for companies looking to develop data and intelligence-driven products and features.
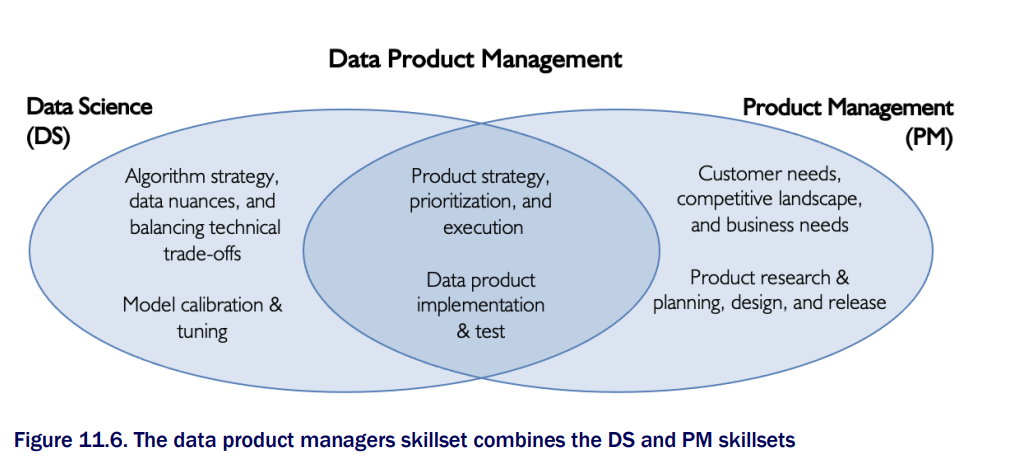
Me ha gustado muchísimo esta sumarización de product management, que es:
>1. What game are we playing?
>2. How do we keep score?